Medical Statistics in Clinical Research – Mean, Median, Standard Deviation, p-value, Chi Squared test
T. Dhasaratharaman*
Statistician, Kauvery Hospitals, India
*Correspondence: Tel: +91 90037 84310 Email: [email protected]
Mean It is also known as an arithmetic mean, or an average. Is very commonly used in papers, so it is important to have an understanding of how it is calculated. It is one of the simplest statistical concepts to grasp.
It is used when the spread of the data is fairly similar on each side of the mid-point, for example when the data are “normally distributed”.
The “normal distribution” is referred to a lot in statistics. It’s the symmetrical, bell-shaped distribution of data (Fig. 1).
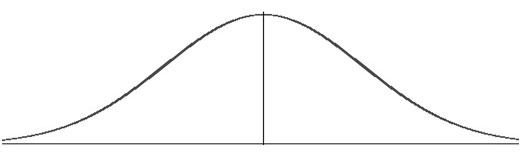
Fig 1. The normal distribution. The centre line shows the mean of the data.
How is it calculated?
The mean is the sum of all the values, divided by the number of values.
Example
Five women in a study on lipid-lowering agents are aged 52, 55, 56, 58 and 59 years.
Add these ages together: 52 + 55 + 56 + 58 + 59 = 280
Now divide by the number of women: 280/5 = 56
So, the mean age is 56 years.
Median
It is also known as Mid-Point. Used in many research papers.
It is used to represent the average when the data are not symmetrical, for instance the “skewed” distribution (not normal distribution) (Fig. 2).
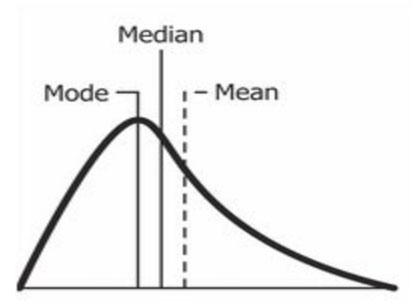
Fig. 2. A skewed distribution. The dotted line shows the median.
How is it calculated?
It is the point which has half the values above, and half below.
Example 1:
Consider the same example from the one used for mean calculation. There are five patients aged 52, 55, 56, 58 and 59, the median age is 56, the same as the mean – half the women are older, half are younger.
However, in the second example with six patients aged 52, 55, 56, 58, 59 and 92 years, there are two “middle” ages, 56 and 58. The median is half- way between these, i.e., 57 years. This gives a better idea of the mid-point of this skewed data than the mean of 62.
Example 2:
A dietician measured the energy intake over 24 hours of 50 patients on a variety of wards. One ward had two patients that were “nil by mouth”. The median was 12.2 megajoules, IQR 9.9 to 13.6. The lowest intake was 0, the highest was 16.7. This distribution is represented by the box and whisker plot.
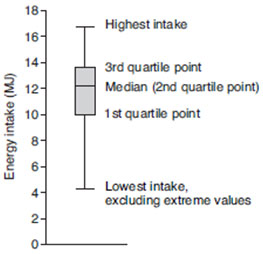
Box and whisker plot of energy intake of 50 patients over 24 hours. The ends of the whiskers represent the maximum and minimum values, excluding extreme results like those of the two “nil by mouth” patients.
Standard Deviation
This is very important concept, Standard deviation (SD) is used for data which are “normally distributed”, to provide information on how much the data vary around their mean.
Interpretation
SD indicates how much a set of values is spread around the average.
A range of one SD above and below the mean (abbreviated to ± 1 SD) includes 68.2% of the values.
±2 SD includes 95.4% of the data.
±3 SD includes 99.7%.
Example 1:
Let us say that a group of patients enrolling for a trial had a normal distribution for weight. The mean weight of the patients was 80 kg. For this group, the SD was calculated to be 5 kg.
±1 SD below the average is 80-5 = 75kg.
1 SD above the average is 80+5 = 85kg.
±1 SD will include 68.2% of the subjects, so 68.2% of patients will weigh between 75 and 85 kg.
95.4% will weigh between 70 and 90 kg (±2 SD).
99.7% of patients will weigh between 65 and 95 kg (±3 SD).
This data correlates to the following graph (Fig. 3).
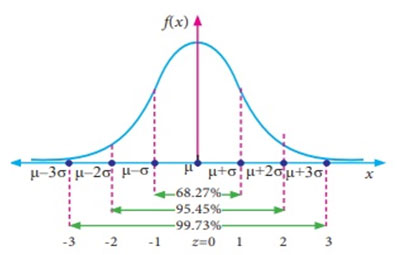
Fig. 3. Graph showing normal distribution of weights of patients enrolling in a trial with mean 80 kg, SD 5 kg.
If we have two sets of data with the same mean but different SDs, then the data set with the larger SD has a wider spread than the data set with the smaller SD.
For example, if another group of patients enrolling for the trial has the same mean weight of 80 kg but an SD of only 3, ±1 SD will include 68.2% of the subjects, so 68.2% of patients will weigh between 77 and 83 kg (Fig. 4).
Fig. 3. Graph showing normal distribution of weights of patients enrolling in a trial with mean 80 kg, SD 5 kg.
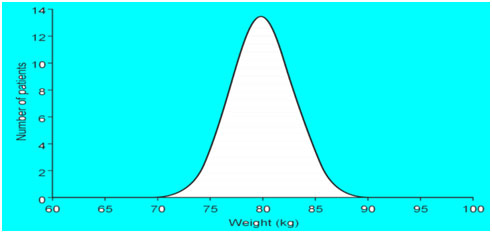
Fig. 4. Graph showing normal distribution of weights of patients enrolling in a trial with mean 80 kg, SD 3 kg.
Example 2:
SD should only be used when the data have a normal distribution. However, means and SDs are often wrongly used for data which are not normally distributed.
A simple check for a normal distribution is to see if 2 SDs away from the mean are still within the possible range for the variable. For example, if we have some length of hospital stay data with a mean stay of 10 days and a SD of 8 days then:
Mean – (2 x SD) = 10 – (2 x 8) = 10 – 16 = -6 days
This is clearly an impossible value for length of stay, so the data cannot be normally distributed. The mean and SDs are therefore not appropriate measures to use.
p-value
The p (probability) value is used when we wish to see how likely it is that a hypothesis is true. The hypothesis is usually that there is no difference between two treatments, known as the “null hypothesis”.
Interpretation
The p-value gives the probability of any observed difference having happened by chance.
p = 0.5 means that the probability of the difference having happened by chance is 0.5 in 1, or 50:50.
p = 0.05 means that the probability of the difference having happened by chance is 0.05 in 1, i.e., 1 in 20.
It is the figure frequently quoted as being “statistically significant”, i.e., unlikely to have happened by chance and therefore important. However, this is an arbitrary figure.
If we look at 20 studies, even if none of the treatments work, one of the studies is likely to have a P value of 0.05 and so appear significant!
The lower the p-value, the less likely it is that the difference happened by chance and so the higher the significance of the finding.
p = 0.01 is often considered to be “highly significant”. It means that the difference will only have happened by chance 1 in 100 times. This is unlikely, but still possible.
p = 0.001 means the difference will have happened by chance 1 in 1000 times, even less likely, but still just possible. It is usually considered to be “very highly significant”..
Example 1:
Out of 50 new babies on average 25 will be girls, sometimes more, sometimes less.
Say there is a new fertility treatment and we want to know whether it affects the chance of having a boy or a girl. Therefore, we set up a null hypothesis – that the treatment does not alter the chance of having a girl. Out of the first 50 babies resulting from the treatment, 15 are girls. We then need to know the probability that this just happened by chance, i.e., did this happen by chance or has the treatment had an effect on the sex of the babies?
The p-value gives the probability that the null hypothesis is true.
The p-value in this example is 0.007. Do not worry about how it was calculated, concentrate on what it means. It means the result would only have happened by chance in 0.007 in 1 (or 1 in 140) times if the treatment did not actually affect the sex of the baby. This is highly unlikely, so we can reject our hypothesis and conclude that the treatment probably does alter the chance of having a girl.
Example 2:
Patients with minor illnesses were randomized to see either Dr XXXX ended up seeing 176 patients in the study whereas Dr. YYYY saw 200 patients (Table 1).
Table 1. Number of patients with minor illnesses seen by two groups
Doctor | Dr. XXXX (n = 200) | Dr. YYYY (n = 176) | p-value |
Patients satisfied with consultation (%) | 186 (93) | 168 (95) | 0.4 |
Mean (SD) consultation length (min) | 16 (3.1) | 6 (2.8) | < 0.001 |
Patients getting a prescription (%) | 58 (29) | 76 (43) | 0.3 |
Mean (SD) number of days off work | 3.5 (1.3) | 3.6 (1.3) | 0.8 |
Patients needing a follow-up appointment (%) | 46 (23) | 72 (41) | 0.05 |
Caution
The “null hypothesis” is a concept that underlies this and other statistical tests. The success of the program is that we have done this for three years continuously before we were confronted with the COVID pandemic.
The test method assumes (hypothesizes) that there is no (null) difference between the groups. The result of the test either supports or rejects that hypothesis.
The null hypothesis is generally the opposite of what we are actually interested in finding out. If we are interested if there is a difference between two treatments, then the null hypothesis would be that there is no difference and we would try to disprove this.
Try not to confuse statistical significance with clinical relevance. If a study is too small, the results are unlikely to be statistically significant even if the intervention actually works. Conversely a large study may find a statistically significant difference that is too small to have any clinical relevance.
Chi Squared test (χ2)
Usually written as χ2 (for the test) or Χ2 (for its value); Chi is pronounced as in sky without the s.
It is a measure of the difference between actual and expected frequencies.
Interpretation
The “expected frequency” is that there is no difference between the sets of results (the null hypothesis). In that case, the Χ2 value would be zero.
The larger the actual difference between the sets of results, the greater the Χ2 value. However, it is difficult to interpret the Χ2 value by itself as it depends on the number of factors studied.
Example
A group of patients with bronchopneumonia were treated with either amoxicillin or erythromycin. The results are shown in Table 2.
Table 2. Type of antibiotic given
Tablet | Amoxicillin (%) | Erythromycin (%) | Total (%) |
Improvement at 5 Days | 144 (60%) | 160 (67%) | 304 (63%) |
No improvement at 5 Days | 96 (40) | 80 (33%) | 176 (37%) |
Over-all | 240 (100%) | 240 (100%) | 480 (100%) |
First, look at the table to get an idea of the differences between the effects of the two treatments. Remember, do not worry about the Χ2 value itself, but see whether it is significant. In this case P is 0.13, so the difference in treatments is not statistically significant.
Caution
Instead of the χ2 test, “Fisher’s exact test” is sometimes used. Fisher’s test is the best choice as it always gives the exact p-value, particularly where the numbers are small.
The χ2 test is simpler for statisticians to calculate but gives only an approximate p-value and is inappropriate for small samples. Statisticians may apply “Yates’ continuity correction” or other adjustments to the χ2 test to improve the accuracy of the p-value.
Journals
- Editorial
- Guest Editorial
- Work stress and fatigue for emergency physicians in the emergency department
- Acute ischemic stroke due to Electrical Injury: A case report
- When the vessels of vessel bleed: A case of aortic intramural hematoma involving descending thoracic and abdominal aorta
- Blunt Injury Abdomen: A case report
- Unveiling the stealth threat: Cervical epidural hematoma
- Comprehensive management of methotrexate poisoning: A Case study and review
- Congenitally corrected transposition of great arteries
- Lemierre’s syndrome
- Nebulized ketamine as a treatment protocol : A case report
- Retroperitoneal hematoma following abdominal aortic aneurysm repair anti-coagulant induced case study and review
- Rupturing Reality
- Severe heat stroke with dyselectrolytemia—intravascular treatment approach
- Temporary heal can possibly kill: A case series on eucalyptus ingestion
- Wellen’s Syndrome
- Editorial
- IMA Meeting March 2024 proceedings
- Burns: Definition and Epidemiology
- Burns management in ER
- Fluid management and wound care in Burn Patients
- Introduction to Burns
- Sepsis management in Burns
- Surgery for Burn patients
- Nutrition care process: In Burns
- EDITORIAL
- EDITORIAL BOARD
- Rare and complex type of MS, tumefactive multiple sclerosis
- Dilemma of decoding a difficult fever
- IMAGE CHALLENGE DIAGNOSTIC IMAGE
- PATIENT’S VOICE – A BOLT FROM THE BLUE
- THE UNIQUE MIND AN ANTHOLOGY OF POEMS
- EDITORIAL
- EDITORIAL BOARD
- SARS-CoV-2 Vaccination Before Elective Surgery
- A guideline for patients with diabetes mellitus, towards self-assessment of their feet
- Evolution of Dashboards and its Effectiveness in Performance Management and Efficient Utilization of Time and Energy
- Understanding and Use of Sensitivity, Specificity, and Predictive Values
- Anorexia Nervosa with Compulsive Exercising – A Case Report
- From the Desk of the Editor-in-Chief
- Section Editor
- PATIENT’S STORY
- The Un-Relished Performance
- THE ELECTRONIC MEDICAL JOURNAL OF KAUVERY HOSPITALS
- Vascular Covid Diaries
- Encephalitis – a success story
- Intracoronary imaging
- Patient’s Voice
- THE CURE BY KAANTHAL MANIKANDAN
- SEIZURE IN A NEWBORN
- SGLT 2 inhibitors in kidney disease
- Editor’s message – Dr Venkita S Suresh
- Preparing your manuscript
- Pulmonary valve endocarditis: a case report
- Upper limb replantations at different anatomical levels: a short case series Kiran Petkar
- Re-challenging asparaginase after cerebral venous sinus thrombosis in 5-year-old with acute lymphoblastic leukemia
- Prevalence of vitamin B12, folate deficiency and homocysteine elevation in ASCVD or venous thrombosis
- HENOCH SCHOENLEIN PURPURA
- Life turns upside down for a young couple and family
- The Humblest Wonder
- Vaccine Induced Cerebro Venous Thrombosis with Thrombocytopenia (VITT) – A Case Report
- Editor-in-Chief
- EDITORIAL
- Neurobiology of Romantic Love
- An interdisciplinary approach to treatment of juvenile OCD: a case report
- Fanconi Anaemia – Need to look at the whole picture
- The Art and Science of Preparation and Publication of Medical Research from Kauvery Hospitals
- FUNDAMENTALS OF STATISTICS
- Diagnostics Images
- A WAKE-UP CALL FROM THE ANAESTHESIOLOGIST, BY DR. VASANTHI VIDYASAGARAN
- A PATIENT’S STORY
- THE FALLACIES OF PERFECTION
- EDITORIAL
- EDITORIAL BOARD
- Difficult to Treat Epilepsy: A Management Primer for Non-Neurologists
- Clinical audit: A Simplified Approachs
- Rationalise and Restrict Antibiotic Use by Utilizing A Proactive Justification Form and Comparing with Earlier Antibiotic Usage in The Same Paediatric Unit in A Tertiary Care Centre
- COVID-19 and Fungal Infection – An Opportune Time: A Case Series
- Thymidine phosphorylase (TYMP) gene stop mutation, G38X, in a familial case of mitochondrial neurogastrointestinal encephalomyopathy
- Image Challenge
- PATIENT’S STORY
- ANTHOLOGY OF POEMS
- POEM
- POEM
- EDITORIAL
- EDITORIAL BOARD
- Hypertension – a renal disease
- A New Cause for Confusion or Concern – A Case Series
- A rare case of acute aortic dissection secondary to a penetrating aortic ulcer in the ascending aorta
- Pacemaker in children – big shoes to fill for small foot
- The Eight Roles of The Medical Teacher – The Purpose and Functions of a Teacher in The Healthcare Professions by Ronald M. Harden & Pat Lilley
- Reducing the stock items – Need to look at the whole picture
- DIAGNOSTIC IMAGES
- Mountain behind a mountain
- The Disappearing Act
- EDITORIAL
- EDITORIAL BOARD
- TO CRUSH OR NOT TO CRUSH? DON’T RUSH TO CRUSH!
- Effectiveness of Pre – Clinical Competency Certification Program on Improving Knowledge of Clinical Practice Among Nursing Internship Students
- Medical Statistics in Clinical Research – Mean, Median, Standard Deviation, p-value, Chi Squared test
- Vertebral artery dissection with thrombosis causing neuralgic amyotrophy
- Atypical Electrical Alternans Due to Large Left Pleural Effusion
- Early presentation of a rare disorder
- DIAGNOSTIC IMAGES
- PATIENT’S STORY
- ANTHOLOGY OF POEMS
- EDITORIAL
- EDITORIAL BOARD
- GUEST EDITORIAL
- Clinical MIS – A Clinical Analyst Review
- Eclampsia and HELLP Syndrome: A Case Report
- Percutaneous Device Closure in A Toddler with PDA and Interrupted IVC
- Pediatric Car Passenger Trauma – A Case Report and A Review of Child Safety Inside a Car
- Use Of Antibody Cocktail, Regn-Cov2, In Two Non-Hodgkin’s Lymphoma (NHL) Patients with Mild Covid-19 Disease, At A Tertiary Care Hospital in South India: A Case Series
- Neurology Update
- Journal scan: A review of ten recent papers of immediate clinical significance, harvested from major international journals
- DIAGNOSTIC IMAGES
- The Patient Is Always Right
- Humanity’s Most Cherished Fiction
- Mucormycosis in COVID-19: A Clinico-Microbiological Dilemma
- ANAEMIA OR HYDROCELE – WHICH SHOULD BE DEALT WITH FIRST
- OUR EXPERIENCE WITH COVID-19 RELATED MUCORMYCOSIS
- DVT in a child: A case to introspect
- Rainbow within a storm
- Reversal of Dabigatran in patients with intracerebral haemorrhage – a narrative review
- STEREOTACTIC BODY RADIOTHERAPY – VT TREATMENT
- TO BOOST OR NOT TO BOOST? INDIAN PERSPECTIVES – COVID VACCINATION
- EDITORIAL
- EDITORIAL BOARD
- GUEST EDITORIAL
- Convalescent Plasma Therapy in Covid-19: A Question of Timing
- High Dose methotrexate in Children with Cancer Without Drug Level Monitoring – 133 Cycles Experience
- Prenatal diagnosis in Thalassemia – Prevention is better than cure
- Improving Outcomes in Children with Cancer – Our Experience
- Autologous Stem Cell Transplantation for Myeloma with CKD
- All Megakaryocytic Macrothrombocytopenia Are Not ITP
- Telomeropathies and Our Experience: A Case Series
- Neurology Update – Neurobiology of Sleep
- Journal scan: A review of twelve recent papers of immediate clinical significance, harvested from major international journals
- The Brush Stroke
- A rare case of flood syndrome: a case report of fatal complication of umbilical hernia in liver cirrhosis
- Editorial
- Kauverian eMedical Journal
- COVID-19 and the Change in Perspective: Musings of Two Seasoned Pediatricians
- World Prematurity Day: Reflections of a Neonatologist
- Epilepsy in Kids: Problems Beyond Seizures
- Overcoming Challenges and Performing First Paediatric Allogenic Bone Marrow Transplantation in Trichy
- Vaccine Induced Cerebro Venous Thrombosis with Thrombocytopenia (VITT) – A Case Report
- DX ICD Implanted After Unmasking Brugada – A Case Study
- DNB Thesis
- Kauvery 4th Annual Nursing Conclave N4 – 2021
- Learning from Experience – 13 and 14
- The Consultation Room
- Journal Scan
- Recommended Reading
- Diagnostic Image
- From Sand to Sky
- Editorial
- Kauverian eMedical Journal
- Transfusion-related acute lung injury
- Equalizing leg length by “lengthening the shorter leg” by surgery: a case report
- VKA induced catastrophic bleeding management with Prothrombin Complex Concentrate (PCC): a practice changer
- Case Report
- Orthopaedics case series
- Morbidity and mortality meetings for improved patient care
- Significance of waist to height ratio as an early predictor in developing metabolic syndrome in children of age group 5-12 years in a tertiary care centre in Trichy: Part IV
- Chapter 15
- The Consultation Room
- Journal scan: A review of 10 recent papers of immediate clinical significance, harvested from major international journals
- Recommended Reading
- Measuring Association in Case-Control Studies
- DIAGNOSTIC IMAGE
- The Stream of Life
- Editorial
- Kauverian eMedical Journal
- Let’s prepare for the unexpected guest who always arrives at odd hours: Pre-eclampsia
- Cerebrospinal fluid-cutaneous fistula after neuraxial procedure and management: a case report
- Impella CP assisted recovery of acute COVID 19 fulminant myocarditis presenting as out of hospital cardiac arrest and cardiogenic shock
- Ischemic stroke after Russel’s Viper snake bite, an infrequent event: a case report
- Nutrition needs of preterm babies
- Chapter 17
- The Consultation Room
- Journal scan: A review of 10 recent papers of immediate clinical significance, harvested from major international journals
- Recommended Reading
- DIAGNOSTIC IMAGE
- Tick Talk
- EDITORIAL
- EDITORIAL BOARD
- Cervical collar in trauma patients – friend or foe?
- Oxygen conservation strategies
- Chronic periodontitis: a case report
- Erythema multiforme in COVID-19: a case report
- Secondary Synovial Chondromatosis of the knee Joint-a case report
- Statistical Risk Ratio (Relative Risk) Data Analysis
- Journal scan: A review of ten recent papers of immediate clinical significance, harvested from major international journals
- Splinter Haemorrhage
- Dignity matters
- Where does time fly to?
- DIAGNOSTIC IMAGE 1
- Editorial
- Editorial Board
- ABO incompatible renal transplant in a post COVID patient with COVID antibodies
- Neuromyelitis Optica early presentation of an adult disorder
- The gamut of neurological disorders associated with COVID-19
- Anaesthetic management of patients with Takayasu arteritis
- My Pains and Gains in Becoming A Doctor
- Significance testing of correlation coefficient
- Journal scan: A review of ten recent papers of immediate clinical significance, harvested from major international journals
- While you were sleeping
- Subliminal Sublimes (Sonnet 1)
- Editorial
- Instructions for Authors
- COVID-19 and mucormycosis: the dual threat
- COVID-19 associated mucormycosis: efforts and challenges
- Saccular abdominal aortic aneurysms: a case series
- Trauma and OCD – A Case of a Boy with Dark Fears
- Chapter 3
- Journal scan: A review of 10 recent papers of immediate clinical significance, harvested from major international journals
- The Consultation Room
- DIAGNOSTIC IMAGE
- A Patient’s Perception of Pulmonology
- Just a leaf
- Editorial
- Editorial Board
- Dilemma of shadows
- One-year journey in Kauvery! Challenges in Neuroanaesthesia and Neurointensive care
- Leadless pacemakers: The future of pacing?
- Clinical Therapeutics: The polymyxins, existing challenges and new opportunities
- Decision to Take Up a Patient in The Presence of Arrhythmias
- Why growing public dissatisfaction about medical profession?
- JOURNAL SCAN
- Diagnostic Image
- The cloud over a young life
- The Waiting Room
- Author Instruction
- Editorial
- Editorial Board
- The French Connection!
- Rare cause of pyrexia of unknown origin: Primary gastrointestinal non-Hodgkin’s lymphoma
- Impact of multi-disciplinary tumour board (MDT) on cancer care
- WPW pathway ablated from uncommon location
- Statistical hypothesis – using the t-test
- Learning from Experience – Chapters 6 and 7
- The Consultation Room
- Journal scan: A review of 10 recent papers of immediate clinical significance, harvested from major international journals
- Diagnostic Image
- When the sun sets over a good life
- The Deepening Dent
- Save Farmers, Save Future
- Editorial
- Editorial Board
- Guest Editorial
- Significance of waist to height ratio as an early predictor in developing metabolic syndrome in children of age group 5-12 years in a tertiary care centre in Trichy: Part I
- Journal Club
- Statistical Non Parametric Mann – Whitney U test
- Learning from Experience – Chapters 8 – 10
- The Consultation Room
- Journal scan: A review of 10 recent papers of immediate clinical significance, harvested from major international journals
- Diagnostic Image
- Valiant Velvet Paws
- Editorial
- Editorial Board
- Repurposing anti-rheumatic drugs in COVID
- An Effective Journal Club Presentation: A Guide
- A Masquerader Vasculitis as Usual: Time Is Tissue
- A Sinister Swelling: A Case Report
- DNB Thesis
- Statistical Regression Analysis
- Learning from Experience – 11 and 12
- The Consultation Room
- Journal Scan
- Diagnostic Image
- Stained not torn
- Editorial
- Author Instruction
- Interventional Nephrology
- Amplified Ears and Listening Brains
- Love makes life worth living
- Usage of Dapagliflozin in Elderly
- Severe Methemoglobinemia Treated Successfully with Oral Ascorbic Acid: A Case Report
- The new imitator
- Liver Cyst
- Learning from Experience – Intra Operative, Chapters 17 and 18
- The Consultation Room
- Journal scan
- Recommended Readings
- Editorial
- Evolution of Emergency Medicine in India and the Emergence of the MEM Program at Trichy
- Welcome to the Dance floor: The Emergency Room
- Life of An Emergency Physician
- A Racing Heart Beat: To Shock or Not
- Being Calm Amidst Chaos: Tips on How to Be an Expert Emergency Nurse
- COVID COVID everywhere, but not a place to run away from!
- Proud to be an Emergency Nurse: Life in the fast lane!!!
- The Journey of a Fresher Nurse in the ED
- Ready, Steady, Go!!! A brief on Green Corridor activation in Organ Transplantation
- An Elevator Story!!!
- Veno-occlusive mesenteric ischemia: A case report
- Stridor: An Alarming Sign in Emergency
- Amnesia in the ER: That Ghajini Moment!!! A Case series
- Survival After Paraquat Ingestion: A Case Series
- When you save one life, you save a family
- Severe Methemoglobinemia, Unresponsive to Methylene Blue
- Severe Meliodosis With Multisystemic Involvement: A Case Report
- A Case Report
- Recognize Rhabdomyolysis early to prevent Acute Kidney Injury and Acute Renal Failure
- New Onset Refractory Status Epilepticus (NORSE)
- Sensitive of EFAST in trauma in correlation with CT scan
- Sub Arachnoid Haemorrhage, management in Emergency and Neuro Intensive care
- Uncommon presentation of Takayasu Arteritis as a convulsive syncope
- All right sided hearts are not Dextrocardia
- COVID and the Salt Story
- Acute Lower Limb Ischemia: A Clue to Underlying Aortic Dissection
- Globe Injury with Orbital Blow Out Fracture
- The heat-stricken life – Treatment in time only saves lives!
- Pneumoperitoneum, does it have any clinical significance?
- Hypertensive Emergency in the ED
- Role of a paramedic in inter-hospital transfer
- Golden Hours in Safer Hands
- Through rough, crowded roads and stagnant waters – we race against time to reach you!
- Editorial
- Author Instruction
- Nana M, et al. Diagnosis and Management of COVID-19 in Pregnancy. BMJ 2022;377:e069739.
- MICS CABG with LIMA and Left Radial artery, harvested by Endoscopic technique: An ultrashort report
- Ultra-Short Case Report
- An interesting case of Bilateral Carotid aneurysms
- A Case Report
- Uterine artery embolization: Saving a mother and her motherhood
- Acute Abdomen – Sepsis – CIRCI: A Success Story
- Diagnostic Image
- Learning from Experience – Intra Operative, Chapters 19 and 20
- The Consultation Room
- Journal Scan
- Recommended Readings
- Editorial
- Kauverian eMedical Journal
- Cardiothoracic surgery in the COVID era: Revisiting the surgical algorithm
- Review
- Corona warrior award
- Case Series
- Takotsubo cardiomyopathy
- Learning from Experience – Chapters 3 and 4
- The Consultation Room – Chapters 41 to 45
- Journal scan
- Recommended Reading
- DIAGNOSTIC IMAGE
- Poem from Staff Nurse
- Editorial
- Author Instruction
- Male V. Menstruation and COVID-19 vaccination
- Efficacy and doses of Ulinastatin in treatment of Covid-19 a single centre study
- Electronic registries in health care
- A case report
- Anaemia in pneumonia: A case report
- Successful treatment of two cases of rare Movement Disorders
- Analysis of variance Two-Way ANOVA
- Learning from Experience – Chapters 5 and 6
- The Consultation Room
- Journal Scan
- Recommended Reading
- Diagnostic Image
- Poem from Staff Nurse
- Editorial
- Kauverian eMedical Journal
- An obituary, farewell to a very dear friend
- ‘Vitamin D’: One vitamin, many claims!
- Implantation of Leadless Pacemaker in a middle-aged patient: An ultra-short case study
- ABO-incompatible renal transplant at ease
- Basal cell adenoma parotid: A case report
- A case report
- Learning from Experience – Chapters 7 and 8
- The Consultation Room
- Journal Scan
- Recommended Reading
- Diagnostic Video
- Poem from Staff Nurse
- Editorial
- March – the Month for Minds to dwell on Multiple Myeloma
- Covid Report
- Research Protocol
- New Arrows in our Quiver, to direct against SARS-CoV-2 variants
- Out-of-hospital cardiac arrest
- Last on the list: A diagnosis seldom considered in males
- Giant T wave inversion associated with Stokes: Adams syndrome
- Learning from Experience – Intra Operative, Chapters 9 and 10
- The Consultation Room
- Definitions of probability
- Journal Scan
- Recommended Reading
- Diagnostic Video
- A Thanksgiving to Cardiac Surgeons
- Editorial
- Kauverian eMedical Journal
- Research Article
- Research Protocol
- Saving the unsavable
- Case Report
- Case Report
- Letters to the Editor
- Diagnostic Image
- Learning from Experience – Intra Operative, Chapters 13 and 14
- The Consultation Room
- Journal Scan
- Recommended Readings
- Editorial
- Author Instruction
- SIGARAM – The Club for Children with Diabetes
- The hope for a better tomorrow
- An unusual complication of polytrauma:
- An enigma at the ER
- Dynamic examination of airway
- Journal Club
- Journal Club
- Journal Club
- Conditional Probability
- Diagnostic Image
- Learning from Experience – Intra Operative, Chapters 15 and 16
- The Consultation Room
- Journal Scan
- Recommended Readings
- EDITORIAL
- Kauverian eMedical Journal
- Junior nurses in Kauvery Hospital on the frontline against the COVID-19 pandemic
- Emergency Medicine, the Emerging Specialty: Leading Light on the entrance to the Health Care Pathway
- Battle of two drugs: Who won? – An unusual presentation
- Return of the native and a resurrected foe: A case of Rhinocerebral Mucormycosis
- Covert invader- atypical presentation of neuronal migration disorder
- Fragile heart: an unusual cause of chest pain
- Goldberger’s ECG sign in Left Ventricular Aneurysm
- Congenital absence of bilateral Internal Carotid Artery: A case report
- The Power of Purple
- Beads of Nature’s Rattle
- Case Report
- Diagnostic Image
- EDITORIAL
- Kauverian eMedical Journal
- Modification of Management Strategies, And Innovations, During SARS Cov2 Pandemic Improved the Quality, Criticality and Outcomes in In-Patients “Rising to the occasion”, the mantra for success in the COVID -19 pandemic
- Time in Range (TIR) In Diabetes: A Concept of Control of Glycemia, Whose Time Has Come
- Kauvery Heart Failure Registry- A Concept
- Shorter Course of Remdesivir In Moderate Covid-19 is as Efficacious as Compared to Standard Regime: An Observational Study
- CASE REPORT
- Lymphoepithelial Carcinoma: A Case Report of a Rare Tumour of The Vocal Cord
- Diabetic Keto Acidosis (DKA), Associated with Failed Thrombolysis with Streptokinase in Acute Myocardial Infarction
- EARNING FROM EXPERIENCE – CHAPTERS 1 AND 2
- The Consultation Room
- Journal scan
- Recommended Reading
- DIAGNOSTIC IMAGE
- Notes to Nocturne
- Editorial
- Kauverian eMedical Journal
- Caring for nobody’s baby
- Special Report
- Research Article
- The curious case of a migrating needle on the chest wall
- Foreign body: A boon at times
- Cardiorenal Syndrome
- What My Grandmother Knew About Dying
- Endovascular Therapy for Acute Stroke with a Large Ischemic Region. N Engl J Med. 2022
- Letter to the Editor
- Clinical outcomes of Coronary Artery Disease in Octogenarians
- Diagnostic Image
- Learning from Experience – Intra Operative, Chapters 11 and 12
- The Consultation Room
- Journal Scan
- Recommended Reading
- colours
- Editorial
- Author Instruction
- Ultrashort Case Report
- Cochlear Implantation: Expanding candidacy and Cost Effectiveness
- Spontaneous Pneumomediastinum in COVID 19 – Tertiary Care Centre Experience in South India
- Amoxycillin Induced Anaphylactic Shock: A Case Report
- An Unusual Cause of Seizures: A Case Report
- Case Report
- Educational Strategies to Promote Clinical Diagnostic Reasoning
- Rheumatic Rarities
- Types of sampling methods in statistics
- Diagnostic Image
- Learning from Experience – Intra Operative, Chapters 21 and 22
- The Consultation Room
- Journal Scan
- Recommended Readings
- Editorial
- Author Instruction
- Journal Scan
- Myeloma
- Posterior Reversible Encephalopathy Syndrome (PRES)
- Prophylactic orthopaedic surgery
- Subclavian steal: An interesting imaging scenario
- Spondylo-epi-metaphyseal dysplasia (SEMD)
- The bleeding windpipe
- Learning from Experience – Intra Operative, Chapters 23 and 24
- The Consultation Room
- Diagnostic Image
- Recommended Readings
- Editorial
- Editorial Board
- Physician, Protect thyself
- Foetal Medicine, the Future is here!
- Japanese Encephalitis: A common menace
- Carpometacarpal dislocation with impending compartment syndrome
- Heterotopic pregnancy
- Femoral Trochantric and Proximal Humerus Fracture, from Diagnosis to Rehabilitation
- My Father’s Heart Block
- EPS page
- CRT CSP Cases
- Journal scan
- Learning from Experience – Intra Operative, Chapters 25 and 26
- The Consultation Room
- Recommended Readings
- Editorial
- Editorial Board
- Multiple Sclerosis: An overview
- A flower born to blush unseen
- Guest Editorial
- Radio-frequency ablation as an effective treatment strategy in a case of VT storm post STEMI
- Lymphatic malformation of tongue
- Bilateral anterior shoulder dislocation in epilepsy: A case report and review of literature
- An unusual cause of Stridor
- Monoclonal Antibodies (mAbs) – the magic bullets: A review of therapeutic applications and its future perspectives
- Write the Talk
- Press release and Comments
- Probability Distribution of Bernoulli Trials
- Journal Scan
- Recommended Readings
- poem-v4i4
- Editorial
- Editorial Board
- Research
- Research
- Lambda-cyhalothrin and pyrethrin poisoning: A case report
- Good Enough
- The Monoclonal antibodies (mAbs): the beginning
- Comprehensive trauma course 2022: Trauma Management & Kauvery
- Comprehensive trauma course 2022: Introduction to Comprehensive trauma course
- Diagnostic Image
- Learning from Experience – Intra Operative, Chapters 27 and 28
- The Consultation Room
- Recommended Readings
- Poem
- Editorial
- The Brave New World of Anaesthesia
- Anesthetic considerations in Wilson’s disease for fess: A case report
- The painful story behind modern anaesthesia
- Anesthesia considerations for Ankyslosing Spondylitis
- Total intravenous anaesthesia: An overview
- Risk stratification for cardiac patients coming for non-cardiac surgeries
- The Anaesthesiologist’s role in fluoroscopic guided epidural steroid injections for low back pain
- Awake Craniotomy
- Malpositioned central venous catheter: Step wise approach to avoid, identify and manage
- Benefits outweighing risk: Neuraxial anaesthesia in a patient with Spina Bifida with operated Meningomyelocele
- Pain free CABG: newer horizon of minimally invasive cardiothoracic surgery a walk through anaesthesiologist perspective
- Stellate Ganglion Block: A bridge to cervical sympathectomy in refractory Long QT Syndrome
- Venous Malformation in Upper Airway – Anesthetic Challenges and Management: A Case Report
- Editorial
- USG guided peripheral nerve block in surgery for hernia
- Anaesthesia and morbid obesity: A systematic review
- Patient-Controlled Analgesia
- Parapharyngeal abscess of face and neck: Anesthetic management
- 3D TEE, a boon for the diagnosis of Left Atrial Appendage thrombus!
- Anaesthetic management of difficult airway due to retropharyngeal abscess and cervical spondylosis
- Expect the unexpected – Breach in continuous nerve block catheter
- Lignocaine nasal spray: An easy remedy for Post Dural Puncture Headache
- Potassium permanganate poisoning and airway oedema
- Angioedema following anti-snake venom administration
- Editorial
- Editorial Board
- Ra Fx ablation of Atrioventricular nodal reciprocating tachycardia
- Pace and Ablate Strategy: Conduction system pacing with AV junction ablation for drug refractory atrial arrhythmia – A novel approach
- Why Cardiac Resynchronization Therapy (CRT) For complete heart block? A case discussion
- Pacemakers and Bradyarrhythmias in Diabetic Mellitus
- Outcomes of Total Knee Arthroplasty in patients aged 70 years and above
- An approach to CBC for practitioners
- Acute cerebral sinus venous thrombosis with different presentations and different outcomes: A case series
- Diet and nutritional care for DDLT: A case study
- Vaccine for Dengue (Dengvaxia CYD-TDV)
- Diagnostic Image
- Learning from Experience – Intra Operative, Chapters 31 and 32
- Recommended Readings
- காவிரித்தாய்
- ATLAS OF HAEMATOLOGY AND HEMATOONCOLOGY
- Editorial
- Editorial Board
- Case reports and Case series:
- Usefulness of NEWS 2 score in monitoring patients with cytokine storm of COVID-19 pneumonia
- Treatment approach for extensively Drug-Resistant Tuberculosis (XDR-TB)
- Modified Lichtenstein mesh repair, for a patient of Coronary Artery Disease, Heart Failure and with Implanted Cardioverter- Defibrillator
- Fever-induced Brugada Syndrome
- Pulmonary Thrombo Embolism: When to Thrombolyse?
- Ultra-Short Case Report
- Learning from the failure of Nebacumab
- Journal scan
- Learning From Experience Intra Operative Chapters 29 and 30
- Recommended Readings
- Poem
- Editorial
- Editorial Board
- Heart transplantation: Life beyond the end of life
- Azithromycin to Prevent Sepsis or Death in Women Planning a Vaginal
- Medial retropharyngeal nodal region sparing radiotherapy versus standard radiotherapy
- Proximie: Patient safety in surgery – the urgent need for reform
- Analysis of femoral neck fracture in octogenarians and its management
- Adult Immunisation in Clinical Practice: A Neglected Life Saver
- “Icing” The Eyes
- Doppler vascular mapping in Arterio Venous Fistula (AVF)
- Cosmesis and cure: Radiotherapy in basal cell carcinoma of the dorsum of nose – A case report
- Pulmonary Hypertension and Portal Hypertension
- Comprehensive review of Drug-Induced Cardiotoxicity
- Statistics – Data Collection – Case Study Method
- Atlas of Haematology and Hematooncology
- PRE-OPERATIVE Chapters 1 and 2 – Learning from Experience
- Chapter 2. Uncertainties in medicine in spite of advances
- No Splendid Child
- Editorial
- Editorial Board
- A Young Girl Lost in the Storm
- ECMO as a bridge to Transplant: A case report
- Renal anemia – from bench to bedside
- Mission Possible
- New kids on the block – Update on diabetic nephropathy therapy
- Infections – Trade off in Transplants
- To Give Or Not to Give – Primer on Bicarbonate Therapy
- Sialendoscopy: Shifting paradigms in treatment of salivary gland disease
- Gait imbalance in a senior due to Chronic Immune Sensory Polyneuropathy (CISP)
- Statistics – Mcnemar Test
- Atlas Of Haematology And Hematooncology
- PRE-OPERATIVE Chapters 3 and 4 – Learning from Experience
- Changing trends a challenge to the already trained
- Journal scan
- Recommended Readings
- EDITORIAL
- EDITORIAL BOARD
- PREGNANCY POST-RENAL TRANSPLANT
- BALLOON-OCCLUDED RETROGRADE TRANSVENOUS OBLITERATION
- REVERSE SHOULDER ARTHROPLASTY FOR ROTATOR CUFF ARTHROPATHY
- THE AMBUSH A TEAM APPROACH
- ENDOSCOPIC TRANS-SPHENOID APPROACH FOR PITUITARY ADENOMA EXCISION
- A STUDY ON PRESENTATION AND OUTCOME OF BULL GORE INJURIES IN A GROUP OF TERTIARY CARE HOSPITALS
- A CASE OF INTERNUCLEAR OPHTHALMOPARESIS AS THE FIRST MANIFESTATION OF MULTIPLE SCLEROSIS
- GRANULOMATOSIS WITH POLYANGITIS AND LUNG INVOLVMENT (WEGENER’S DISEASE)
- RITUXIMAB (RITUXAN, MABTHERA) IN THE TREATMENT OF B-CELL NON-HODGKIN’S LYMPHOMA
- STATISTICAL SIGNIFICANCE
- Atlas Of Haematology And Hematooncology
- PRE-OPERATIVE CHAPTERS 5 AND 6 – LEARNING FROM EXPERIENCE
- Chapter 4: Diagnostic process often reversed
- Journal scan: A review of 25 recent papers of immediate clinical significance, harvested from major international journals
- Recommended Readings
- ஆரோக்கியம் நம் கையில்
- வெற்றியின் பாதை
- Editorial
- Editorial Board
- Prevalence of vitamin D deficiency in a multi-speciality hospital orthopaedic outpatient clinic
- Esomeprazole induced Hypoglycemia
- Dynamic external fixator for unstable intra articular fractures of Proximal Interphalangeal Joint (PIP): “Suzuki” frame
- How to Practice Academic Medicine and Publish from Developing Countries? A Practical Guide, Springer Nature, 2022
- WINTNCON 2022 – Scientific Program
- Pulmonary Thrombo Embolism: A state of the art review
- Role of Artificial Intelligence in improving EHR/EMR and Medical Coding and billing
- Monoclonal Antibodies: Edrecolomab and Abciximab
- Atlas of haematology and hematooncology
- Journal scan
- Learning from Experience – Intra Operative, Chapters 31 and 32
- What doctors must learn: Doctor, look beyond science
- Recommended Readings
- I Whisper Secrets In My Ear
- EDITORIAL
- Instructions for Authors
- Mismatched Haploidentical Bone Marrow Transplantation in a 10-year-old boy with relapsed refractory acute lymphoblastic leukemia, at Trichy
- ST-Segment Elevation is not always Myocardial Infarction
- Acyanotic Congenital Heart Disease, repaired, evolves into a Cyanotic Congenital Heart Disease and presents with an atrial tachycardia
- Family medicine – caring for you for the whole of your life. A Lost and Found Art
- The Principles and Practice of Family Medicine
- Complete Heart Block
- Sick Sinus Syndrome (SSS)
- The First Ever National Award Comes to Kauvery Hospital Chennai & Heart City for Safety and Workforce Category in IMC RBNQA Milestone Merits Recognition 2022
- Diagnostic Image
- PRE-OPERATIVE CHAPTERS 7 AND 7 – LEARNING FROM EXPERIENCE
- Chapter 5: Super-specialist – boon or bane
- Journal scan: A review of 42 recent papers of immediate clinical significance, harvested from major international journals
- RECOMMENDED READINGS
- நேரம் ஒதுக்கு
- மருத்துவரின் மகத்துவம்
- EDITORIAL
- Instructions for Authors
- Surgical Management of Covid-19 Associated Rhino-Orbito-Cerebral-Mucormycosis (Ca-Rocm) – A Single Centre Experience
- Knee Joint Preservation Surgeries
- Guest Editorial Comments
- Ventricular Septal (VSR) closure with ASD device
- Newer Calcium Debulking Angioplasty technique of Orbital Atherectomy
- An hour-long CPR to restart the heart
- VT or SVT with aberrancy?
- Pituitary Neuroendocrine Tumor (PitNET)
- VSD Device Closure
- PDA Device Closure
- Iron Deficiency Anemia, Post MVR
- Torsades de Pointes
- Quality improvement project to Reducing the Malnutrition Rate of ICU patients from 43% to 20%
- Long term use of Amiodarone in Cardiac patients: A Clinical Audit
- Statistical Independent Events and Probability
- PERI-OPERATIVE Chapters 9 and 10 – Learning from Experience
- Journal scan: A review of 40 recent papers of immediate clinical significance, harvested from major international journals
- EDITORIAL
- Kauverian Medical Journal
- First da Vinci Robotic Surgery in Carcinoma Prostate: A Case Report
- Black burden or Taylor the saviour: A case report
- Analysis of differences in Oncology practice between the United Kingdom and India
- A Case of Takayasu Arteritis
- Idiopathic Dilated Pulmonary Artery (IDPA)
- ECG Atlas
- Unusual cause of Dysphagia: A case report
- Tu Youyou: The scientist who discovered artemisinin
- Continuing Nursing Education (CNE) on Risk assessment tools, to assess vulnerable patients at Kauvery Hospital, Tennur
- DIAGNOSTIC IMAGE
- PRE-OPERATIVE CHAPTERS 11 AND 12 – LEARNING FROM EXPERIENCE
- OLD AND NEW – MAKE THE BEST OF THE TWO
- Journal scan: A review of 30 recent papers of immediate clinical significance, harvested from major international journals
- Recommended Readings
- EDITORIAL
- INSTRUCTION TO AUTHORS
- A pregnant patient with DKA, septic shock and a lactate mystery
- Radical Thymectomy in Myasthenia Gravis through Partial Sternotomy approach: A report on three patients
- In-utero blood transfusion in two etiologically distinct anaemic fetus
- RARE CAUSE OF PULMONARY HYPERTENSION
- Acute Rheumatic fever is still an enigma
- A remarkable journey: Managing LQTS in a 43-year-old female with recurrent syncope and seizure
- An unusual case of Acute Coronary Syndrome
- Reversible cause of severe LV Dysfunction in Left Bundle Branch Block
- A case study on Rhino-Orbital-Cerebro- Mucormycosis
- Snakebite and its management
- Total Elbow arthroplasty in Post Traumatic arthritis
- Wellens Syndrome: An ECG finding not to miss!
- Dr. C.R. Rao Wins Top Statistics Award a look back at his pioneering work
- PRE-OPERATIVE Chapters 13 and 14 – Learning from Experience
- Chapter 7. Doctor-patient relationship
- Journal scan: A review of 30 recent papers of immediate clinical significance, harvested from major international journals
- EDITORIAL
- INSTRUCTION TO AUTHORS
- FROZEN ELEPHANT TRUNK (FET) PROCEDURE IN A 52 YEARS OLD CHRONIC AORTIC DISSECTION PATIENT
- DIAGNOSING AND MANAGING EISENMENGER SYNDROME IN A YOUNG MALE
- STEMI EQUIVALENT BUT STEMI!
- TEMPORARY HEAL CAN POSSIBLY KILL!
- UNDERSTANDING PUBERTY
- THE IMPORTANCE OF STATISTICS IN HEALTHCARE
- JOURNAL SCAN: A REVIEW OF 44 RECENT PAPERS OF IMMEDIATE CLINICAL SIGNIFICANCE, HARVESTED FROM MAJOR INTERNATIONAL JOURNALS
- DIAGNOSTIC IMAGE
- PRE-OPERATIVE CHAPTERS 15 AND 16 – LEARNING FROM EXPERIENCE
- CHAPTER 8. DOCTOR-DOCTOR RELATIONSHIP
- EDITORIAL
- GUEST EDITORIAL
- CLINICAL AUDIT: AN INTRODUCTION
- YOUNG ACS AUDIT
- CLINICAL AUDIT: ANAESTHESIA
- CLINICAL OUTCOME IN ICU PATIENTS
- RATE OF MALIGNANCY IN INDETERMINATE OVARIAN CYST – A PROCESS AUDIT
- PATTERNS OF NEEDLE DISPOSAL AMONG INSULIN USING PATIENTS WITH DIABETES MELLITUS: AN AUDIT
- CONTINUOUS PATIENT MONITORING – LIFE SIGNS
- AGE IS JUST A NUMBER!
- SVT WITH LEFT BUNDLE BRANCH BLOCK FOLLOWING GASTRECTOMY
- COMPLEX AORTIC DISSECTION WITH MULTIFACETED CLINICAL PRESENTATIONS
- EDITORIAL
- POST-OPERATIVE SORE THROAT IN GA: A CONCERN
- NEONATAL HLH: OUR EXPERIENCE
- CLINICAL REVIEW MEET 16TH NOV 2023: CHALLENGES IN SETTING UP A NEW HSCT CENTRE IN A TIER-2 CITY IN INDIA
- CARDIAC BIOMARKERS: CLINICAL UTILITY
- HIRAYAMA DISEASE: A CLINICAL-RADIOLOGICAL REVIEW
- ECG AS A WINDOW OF OPPORTUNITY: FOR HYPERKALEMIA
- EFFECTIVENESS OF ROOD’S APPROACH BASED PAEDIATRIC OCCUPATIONAL THERAPY MANAGEMENT: ON CHILDREN WITH CONGENITAL MUSCULAR TORTICOLLIS
- ELTROMBOPAG, A NOVEL THROMBOPOIETIN (TPO) RECEPTOR AGONIST: AN OVERVIEW
- HENOCH-SCHONLEIN PURPURA
- SUBMANDIBULAR GLAND SIALADENITIS SECONDARY TO SUBMANDIBULAR CALCULUS
- LEAN HOSPITALS
- STATISTICS BLACK-SCHOLES MODEL
- PERI-OPERATIVE CHAPTERS 17 AND 18 – LEARNING FROM EXPERIENCE
- CHAPTER 9. BUILDING BLOCKS OF PATIENT CARE
- JOURNAL SCAN: A REVIEW OF 30 RECENT PAPERS OF IMMEDIATE CLINICAL SIGNIFICANCE, HARVESTED FROM MAJOR INTERNATIONAL JOURNALS
- EDITORIAL
- INSTRUCTIONS TO AUTHORS
- ROTATHON-SERIES OF SUCCESSFUL ROTA CASES LAST 2 MONTHS: AN AUDIT
- OUTCOMES OF AKI AUDIT IN KAUVERY
- ETIOLOGY, CLINICAL CHARACTERISTICS AND OUTCOMES OF PATIENTS WITH ACUTE PANCREATITIS IN KAUVERY CANTONMENT HOSPITAL (KCN), TRICHY
- USE OF BLOOD PRODUCTS AND STEROIDS IN THE MANAGEMENT OF DENGUE AT KAUVERY TRICHY HOSPITALS: A CLINICAL AUDIT
- A CLINICAL AUDIT: ON THE MANAGEMENT OF ECTOPIC PREGNANCY
- DIABETIC KETOACIDOSIS WITH HIGH ANION GAP METABOLIC ACIDOSIS
- HYPOKALEMIC PARALYSIS FROM DISTAL RENAL TUBULAR ACIDOSIS (TYPE-1)
- DELAYED PRESENTATION OF INTERMEDIATE SYNDROME IN A PATIENT WITH ORGANOPHOSPHORUS POISONING: A CASE REPORT
- SCAPULA FRACTURES: DO WE NEED TO FIX THEM?
- SPONTANEOUS CSF RHINORRHEA
- DANCING WITH DIABETES: AN UNUSUAL CASE OF CHOREA HYPERGLYCEMIA BASAL GANGLIA SYNDROME
- CONVALESCENT RASH OF DENGUE
- ECG ATLAS 2
- MI MIMICKER
- PERFORATION PERITONITIS-A CASE REPORT
- APPLICATION OF EVIDENCE BASED PRACTICE CARE FOR INDIVIDUALS WITH SPINAL CORD INJURY WITH FUNCTIONAL DIFFICULTIES: ROLE OF THE OCCUPATIONAL THERAPY PRACTICE GUIDELINES
- METHOTREXATE INDUCED MUCOSITIS AND PANCYTOPENIA: A CONSEQUENCE OF MEDICATION ERROR IN A PSORIASIS PATIENT
- POST OPERATIVE CHAPTERS 1 AND 2 – LEARNING FROM EXPERIENCE
- CHAPTER 10. NURTURING SELF
- விடாமுயற்சி வெற்றி தரும்
- JOURNAL SCAN: A REVIEW OF 26 RECENT PAPERS OF IMMEDIATE CLINICAL SIGNIFICANCE, HARVESTED FROM MAJOR INTERNATIONAL JOURNALS
- EDITORIAL
- EDITORIAL BOARD
- LIMB SALVAGE IN EXTREMITY VASCULAR TRAUMA: OUR EXPERIENCE
- MANAGEMENT OF URETHRAL CATHETER RELATED PAIN IN RENAL TRANSPLANT RECIPIENTS: A CLINICAL AUDIT
- NO PAIN VEIN GAIN WITH PRILOX IN PAEDIATRIC POPULATION
- GUIDELINE-DIRECTED MEDICAL TREATMENT (GDMT) OF HEART FAILURE AT KAUVERY HOSPITALS: A CLINICAL AUDIT
- PATIENTS STORY: DIGNITY MATTERS
- PATIENTS STORY: DIGNITY MATTERS
- VIDEO PRESENTATION- CT CORONARY ANGIOGRAPHY
- DRUG INDUCED HYPERKALEMIA
- ADULT NEPHROTIC SYNDROME
- EXPLORING COMPLEX CARDIAC CASES: INSIGHTS FROM DIVERSE PRESENTATIONS IN MID-AGED WOMEN
- EFFECTIVENESS OF ACTIVITY CONFIGURATION APPROACH BASED OCCUPATIONAL THERAPY INTERVENTION FOR CHILDREN WITH CONGENITAL MUSCULAR TORTICOLLIS: A CASE STUDY
- CARDIOMYOPATHY AND ITS ECHO FINDINGS
- THE STRESS TEST ON THE TREADMILL
- POST OPERATIVE CHAPTERS 3 AND 4 – LEARNING FROM EXPERIENCE
- CHAPTER 11. BALANCE IN LIFE BEYOND BANK BALANCES
- JOURNAL SCAN: A REVIEW OF 30 RECENT PAPERS OF IMMEDIATE CLINICAL SIGNIFICANCE, HARVESTED FROM MAJOR INTERNATIONAL JOURNALS
- காணும் பொங்கலும் மனித மன மாற்றமும்
- EDITORIAL
- INSTRUCTIONS TO AUTHORS
- PREVALENCE OF STREPTOCOCCUS PNEUMONIAE SEROTYPES IN AND AROUND TRICHY AND ITS CLINICAL RELEVANCE
- ANAESTHETIC MANAGEMENT OF A PATIENT WITH HUGE GOITER: A CASE REPORT
- EUGLYCEMIC DIABETIC KETOACIDOSIS A RARE CAUSE FOR DELAYED EXTUBATION
- SUCCESSFUL PREGNANCY IN ASD PATIENT – COMPLICATED BY SEVERE PULMONARY ARTERIAL HYPERTENSION
- ENDOCRINE COMPLICATION OF GROWTH HORMONE SECRETING TUMOR: A CASE REPORT
- EFFICACY OF EARLY DIAGNOSIS AND DEVELOPMENT APPROACHES OF PAEDIATRIC OCCUPATIONAL THERAPY ON ACUTE DISSEMINATED ENCEPHALOMYELITIS: A CASE REPORT
- POST OPERATIVE CHAPTERS 5 AND 6 – LEARNING FROM EXPERIENCE
- CHAPTER 12. MILES TO GO – BEFORE TEACHING BECOMES LEARNING
- JOURNAL SCAN: A REVIEW OF 26 RECENT PAPERS OF IMMEDIATE CLINICAL SIGNIFICANCE, HARVESTED FROM MAJOR INTERNATIONAL JOURNALS
- காவேரி – 25
- POEM – மண்ணில் பிறந்தது சாதிப்பதற்குத்தான்
- Editorial
- Video – Left main disease: Is it still a Surgeons Domain
- Propofol and the unspoken pain
- Thrombocytopenia in pregnancy: Experience from a tertiary care centre of a tier 2 city in South India
- Snake envenomation and its managements
- Essentials of Advanced Cardiac Life Support
- Video – Sepsis Management
- The phantom Block: Ogilvie’s
- Airway Management in an Adult with Subglottic Stenosis: Overcoming Ventilation Challenges with a Small Endotracheal Tube ”
- Descending thoraco-abdominal aortic aneurysm rupture with left massive hemothorax –diagnosis and emergency management
- C-IIH (Chronic IIH Imaging in Headache) study: Hospital-based pictorial review of Chronic IIH variants Neuroradiologist’s perspective
- Diagnostic Image: Small bowel diverticulosis
- Occupational therapy interventions for Schizophrenia: A systematic review
- Post-Operative Chapters 23-25
- Chapter 21. How to communicate need for further tests or referral for second opinion?
- Journal scan: Journal scan: A review of 13 recent papers of immediate clinical significance, harvested from major international journals
- Poem- கருணையின் வடிவமே தானம்
- Poem – நீ உன்னையறிந்தால்
- EDITORIAL
- EDITORIAL BOARD
- Audit Appraisal: An overview
- Intra Ventricular antibiotics: Our experience
- Transfusion Reactions: A clinical audit
- Postoperative pain management
- Rising concerns of Carbapenem resistant Enterobacteriaceae and the options available: An overview
- Ultrashort case report on VF storm in immediate Post CABG Period—‘Angry Purkinje Syndrome’
- Lynch syndrome, diagnostic challenges and management: A case report
- Bilateral posterior shoulder fracture – dislocation due to seizure, an uncommon injury: A case report
- Navigating amlodipine overdose: A multi-disciplinary approach in a 23 year old female
- Exploring the interplay between sleep patterns and behavioural characteristics in individuals with Autism Spectrum Disorder (ASD)
- POST OPERATIVE Chapters 7 and 8 – Learning from Experience
- Chapter 13. Art of communication and counselling
- Journal scan: A review of 20 recent papers of immediate clinical significance, harvested from major international journals
- மருத்துவமனையின் தேவதையே
- மருத்துவ சிந்தனை விருந்து
- Editorial
- Instructions for Authors
- Cardiac surgery in an octogenarian Indian population
- Mycotic Aortoiliac Aneurysm: A case series
- The Scorpion Block—A Sting operation
- Arterial Thoracic Outlet Syndrome: A case report
- Infective endocarditis in adult congenital heart disease: A case report
- Takotsubo cardiomyopathy: A case report
- Cerebral Venous Thrombosis (CVT): A case report
- Hemophagocytic Lymphohistiocytosis in Dengue
- A prospective observational study on the prescription of Guideline Directed Medical Treatment (GDMT) for Heart Failure at Kauvery Heart City
- Guideline-Directed Medical Treatment (GDMT) of Heart Failure at Kauvery Hospitals: A clinical audit
- POST OPERATIVE Chapters 9 and 10 – Learning from Experience
- Chapter 14. How to keep updated in busy medical practice?
- Journal scan: A review of 15 recent papers of immediate clinical significance, harvested from major international journals
- சிந்தனையே சிறப்பு
- தோல்வியே வெற்றியின் பாதை
- Editorial
- Results of Mechanical Thrombectomy in acute stroke: A case study in a Tier 2 city in India
- A clinical audit—cost effective way of managing superficial chest wound infections post sternotomy
- Dental implants: Our experience and expertise
- A non-randomized retrospective study on the management of pediatric septic arthritis
- Analysis of femoral neck fracture in octogenarians and its management
- Airway management of a huge thyroid: A case report
- Cardiac Arrhythmias in the ER setting
- Effectiveness of tactile-spatial oriented approach based paediatric occupational therapy intervention for children with Infantile Hemiplegia
- Corporate citizenship in healthcare: Nurturing social responsibility
- Guillain-Barré syndrome: A case report
- POST OPERATIVE Chapters 11 and 12 – Learning from Experience
- Chapter 15. How to be rational in practice?
- Journal scan: Elsevier’s Medicine, Current Issue, Volume 52 Issue 6, June 2024, Seminar on Poisoning
- Recommended Readings
- இயற்கை சொல்லும் செய்தி
- Editorial
- Instructions for Authors
- A rare organism causing septic arthritis of hip joint
- ICD implant in 6 year old with Jervell and Lange-Nielsen (JLN) syndrome
- A clinical audit on Bariatric/Metabolic surgery
- Clinical audit on Nil per Oral (NPO)
- A non-randomized clinical study on heat stroke
- Stress cardiomyopathy with posterior reversible encephalopathy syndrome in Eclampsia
- Intro-Reversible Cerebral Vasoconstriction Syndrome (RCVS): An ultra-short case report
- A 2 am Tamponade: Critical call to action
- Immune Checkpoint Inhibitors, and in combination with JAK inhibitors: The modelling for the future
- Optimizing the patient journey in hypertrophic cardiomyopathy—Patient and clinician perspectives: Summary of CME from Medscape
- ER Intervention
- Diagnostic Images/Videos
- A randomized controlled trial: Different types of blinding
- Journal scan – June
- Journal scan – July
- Post-Operative Chapters 13, 14
- Chapter 16. How to enjoy medical practice?
- Driving business growth with 5S and lean strategies: A transformative session for corporations in the Trichy region
- Poem: 25 – வருடங்களாக காவேரி
- Editorial
- A Data Audit: Tool for Good Clinical Practice
- Ortho rehab audit at Kauvery Hospital, Trichy
- Endovascular Thrombectomy in young patient with a stroke – surgical procedure decompressive craniotomy
- Secondary HLH (Hemophagocytic Lymphohistiocytosis): An ultra-short case report
- Prone ventilation: A case series
- Dermatologic Emergencies: A case series
- Managing DKA at the ER
- Podcast – Managing DKA at the ER
- Insertion of tunneled hemodialysis catheter through the collateral vein adjacent to the thrombosed right jugular vein.
- Access failure with dysfunctional tunnelled haemodialysis catheter in the presence of exit-site infection
- Video – A multidisciplinary approach to preventing sudden cardiac death
- Dasatinib in pediatric Chronic Myeloid Leukemia: An overview
- Dyslipidemia management: Indian perspective
- Pharmacological stress testing: Assessing heart health without exercise
- Journal scan: A review of 15 Ethics with clinical significance, harvested from a major international journal
- Post-Operative Chapters 15 and 16
- Chapter 17. How to find time for the family
- Poem – உனக்காக நீ
- Editorial
- An audit: A silent disease-Osteoporosis
- An approach to antibiotic therapy: A primer for all specialties
- Audit on pediatric Cholesteatoma
- Clinical audit: ER to ICU transit time
- A case report on Multiple myeloma: Value of pre and post-treatment MRI
- Unrelated donor bone marrow transplantation in 3 year old with Acute Myeloid Leukemia
- Infective endocarditis: A case series
- A Retrospective observational study on Scrub typhus paediatric population in Kauvery Hospitals, Trichy
- Clinical Pharmacy Approach: To a patient on multiple anticonvulsants for “Difficult to control’’ seizure disorder
- An analysis of the Urea to Creatinine Ratio (UCR) in different cardiac surgeries
- Journal scan – 1
- Journal Scan – 2
- Post-Operative Chapters 17 and 18
- Chapter 18. How to be legally safe in medical practice?
- Poem – காவேரியின் மாரத்தான்
- Poem – ஆரோக்கியம் என்னுடையது
- Editorial
- Clinical audit on antibiogram
- Renal transplantation in marrow dysfunction: A case series
- Empowering lifesavers: Impact of BLS and PALS training for PICU and critical care nurses
- Myotonia congenita: A case series
- Utilization of Inj. Sovateltide: A novel injectable formulation
- Retroperitoneal sarcoma: A report of two cases
- Traumatic simultaneous bilateral femur and tibia shaft fracture in an 8-year-old male child: An unusual case report
- Echocardiography in the assessment of shock
- Plasma combats angioedema: A case report
- A case report and video presentation on a 9-year-old boy who underwent PAPVC
- Interventional Radiology: Large gastric varices
- Interventional Radiology: A case of active PV bleeding post-D and C day 12
- Diagnostic Images: Exencephaly
- A short case report on Hyperdontia (extra teeth)
- Post-Operative Chapters 19 and 20
- Chapter 19. How to deal with incurable disease?
- CII CFO Excellence award: A recognition of exceptional leadership
- Journal scan
- Poem – மறுக்காதே மறவாதே
- Editorial
- An audit: Microvascular Free Flaps
- DSME Programs – Lessons learned
- Surgical Reconstruction Of Acromio Clavicular Joint Dislocation Using Double Endobutton – A Case Report
- Complex Iliac Artery And Venous Reconstruction In Case Of Polytrauma – Case Report
- Basics of statistics- from of a clinician’s perspective
- A comprehensive review on Rabies: Clinical aspects and treatment
- Lateral Medullary Syndrome – Case Report
- Postpartum ischemic stroke – case report
- Proning Saves Lives
- Video – A challenging case of Recurrent craniopharyngioma with supra and infra tentorial cyst and hydrocephalus
- Event: Update on Atrial Fibrillation
- Investigating the Role of Activity-Based Configurational Developmental approach in Managing Mitochondrial Leukodystrophy in Children
- Post-Operative Chapters 21 and 22
- Chapter 20. How to deal with failure of treatment
- Journal scan: A review of 12 recent papers of immediate clinical significance, harvested from major international journals
- Poem – தன்னம்பிக்கையும் விடாமுயற்சியும்
- Extraordinary Edition of the Kauverian